An Outsiders Inside view of the NHL Stats Revolution – Part 1
By Kent Wilson
9 years ago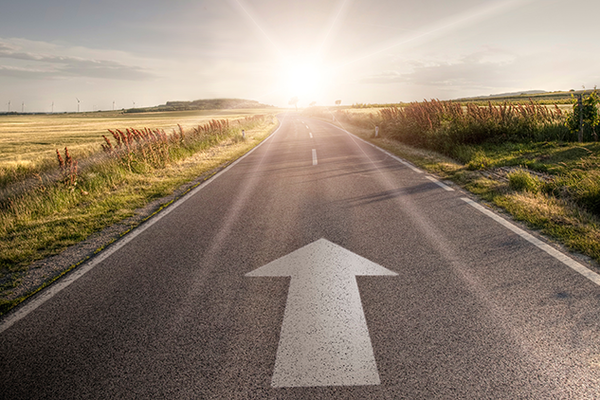
I began writing about hockey in 2005. Through a combination of timing and proximity, I have had the fortune of a ringside view of the genesis, dissemination and popularization of hockey’s so-called advanced stats. Over this two part series, I will share some of the insights engendered by this somewhat unique perspective. My focus will be on what’s currently happening in the league now as teams flock to build analytic departments around possession theory, as well as why the movement grew outside of the league’s front offices and where we may expect this sort of analysis to go in the future.
The off-season of 2014 may well be remembered as the summer of stats, although corsi numbers and their various accoutrements made their way into popular discourse earlier in the year when they began popping up in national broadcasts and game day discussions. No doubt the new numbers began to spread in part due to the spectacular failure of the Toronto Maple Leafs, a club that had been deemed as a bellwether for possession-based theory at the onset of the season. Their subsequent 84-point, 12th place finish in the face of expanded expectations and executive confidence was the metaphorical canary in the coal mine as it were.
The Leafs case study also seems to be the main impetus behind the recent rash of stats-nerd hires by NHL teams. Lead by the Leafs acquisition of Kyle Dubas, Darryl Metcalf (Extra Skater), Cam Charron and Rob Pettapiece, a wave of official interest has crashed over the shores of the auto didactic amateurs who were instrumental in spurring the movement, subsequently washing advanced stats further inland into public consciousness by giving the numbers an imprimatur of authority that was previously lacking.
From my own knowledge base and observations as well as discussions with NHL teams and stats hires, here’s a general road map for what is – or, at least, what should be – occurring in the new analytics departments springing up around the league.
Establishing Roles
It’s no doubt a mistake to claim that “analytics” is new to NHL clubs. Indeed, no doubt every team already had at least one executive and a handful of interns (or healthy scratches) busily collecting internal metrics, watching video and making recommendations. What’s new, instead, is an understanding and adherence to 1.) the work and theory that underpins corsi and related measures and 2.) the collection of skills and abilities required to conduct true “big data” statistical research.
As such, the emerging blueprint for the evolution of NHL analytics departments is hinted at by the Toronto Maple Leafs hires: a collection of individuals with related, but differing, skill sets that, when combined, can theoretically perform rigourous empirical studies and create valid statistical models. The interdependent roles roughly translates to:
1.) Lead Analyst(s)
A person or persons with deep background knowledge of the existing work and theory in the field, as well as hockey in general and the team in question. This role would involve filtering through both qualitative and quantitative information to conjure insights about individual players, trends in player valuation, on-ice tactics and exploitable inefficiencies in the market.
Deep existing knowledge would mean not replicating work or investigating previously abandoned blind alleys. A familiarity with behavioral economics and probabilistic thinking would be a strong requirement of the role since it requires big picture, “forest” (rather than “trees”) thinking and an ability to resist many of the common psychological pitfalls that tend to skew decision making in the NHL (and many other human endeavours) currently.
2.) Computer Scientist
Building a robust internal dataset requires the creation of databases, stats counting applications and user interfaces. Teams should be looking for a computer scientist who is not only capable of building spreadsheets, but also one familiar with what has worked “in the wild” already. Although this is a task that could be contracted out to any moderately skilled developer, the need to educate someone from scratch about what is important in hockey in general and what is important in advanced stats specifically could result in a lot of wasted time and work.
3.) Math man
Though the lead analyst(s) should be conversant in statistical concepts and modelling, it may may make sense to parse this particular set of skills into a separate role entirely. Having a deep background in statistical theory and modeling would ensure the potential insights rendered from data collection are mathematically valid.
4.) Champion
Perhaps the most important role is that of the analytics champion. With these departments suddenly being grafted on to existing appendages in an organization, there is no small threat of the “stats guys” being segregated behind a thick, sound proof wall of internal ambivalence or apathy. Particularly since some of the insights of corsi-based theory tend to fly in the face of hockey orthodoxy.
If corsi concepts and strategies are to trickle up into the front office or down into on-ice tactics, a club will likely need at least one stakeholder who is willing to advocate for their validity and utility.
Low Hanging Fruit
Besides the fact that the NHL is a “me-too” league, there are practical reasons for teams to be jumping on the bandwagon sooner rather than later (with apologies to the few clubs that were ahead of their time). The two most notable are:
1.) Pluck the low hanging fruit
2.) Inoculate against opponents gaining a competitive advantage
It’s true that hockey’s fancy stats are still very much in their infancy. Though not perfect, the theory is at least developed enough to help decision makers be less wrong even as theory matures. We might one day get closer to being more predictive with more precision, but corsi can help GM’s avoid landmines, make slightly better bets on young players or understand when their roster construction is fundamentally flawed right away. If integrating this sort of knowledge can help a team avoid just a single damaging, albatross contract in the realm of David Clarkson or Brooks Orpik, it will pay for the entire first 5 years of the departments existence. At least.
The second and more cogent issue is the possibility of falling behind other clubs in terms of statistical understanding and tools, both in perception and reality. It could be rather damaging for a GM to be taken to the cleaners in a few trades by clubs with known stats departments, for instance*.
*(Aside – I suspect Tyler Bozak will be put on the trade block any day now. Expect him to be offered to the clubs who haven’t yet bought into possession theory first)
The Ideal State
There is obviously a long road ahead in terms of new stats actually having an impact at the NHL level. Though they have infiltrated analysis on mainstream websites and broadcasts to some degree and there have been a few high profile blogger hires so far, it’s a different matter entirely for them to hold any sway in the show.
The ideal state for advanced stats advocates and organizations alike is to build understanding of current and legacy corsi theory; conduct internal processes to gain proprietary data/unique knowledge, and; find a way to marry these things with practical applications and the existing knowledge base in the NHL.
Each step along the path is no small feat in isolation and I expect we’ll see some clubs fail at one or all of them before finding their footing (or abandoning the experiment altogether). For those who get it right, the sweet spot will be intersection of math, empiricism and real life experience, as expressed by this graphic (grabbed from Nassim Taleb’s Facebook page):
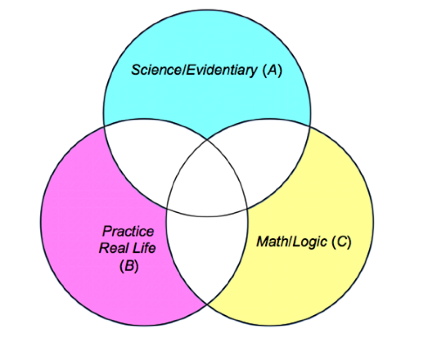
NHL organizations mostly have the left bubble wrapped up. Their challenge now is to develop the other two spheres and integrate them accordingly.
Next up – The reasons why corsi grew up outside of the NHL and where it’s going next
Recent articles from Kent Wilson